Dr. Inessa Seifert is Co-Chair of the Working Group „AI at the Edge“ at EPoSS. Here she answers three questions about this technology.
What is Edge AI and what role does it play with regard to smart systems?
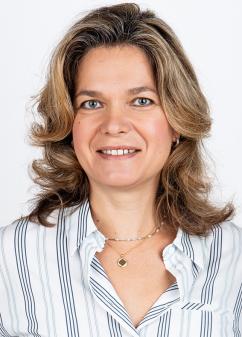
"By definition, edge computing infrastructure is located in a local data centre or on a portable device, not remotely in the cloud. The concept of edge computing can only be understood in the context of the cloud. Cloud-edge-IoT systems are complex infrastructures where data and information processing takes place not only in the cloud, but also on local devices.
Edge AI applications are diverse, ranging from health analysis on smart devices such as watches, or the use of flexible electricity tariffs by washing machines, to predictive maintenance of production lines and wind turbines. Such systems require specialised hardware and software components that enable data processing, analysis and AI reasoning at the edge.
There is a lot of overlap with intelligent systems. As complex systems, intelligent systems require the integration of different components. These components may be, for example, sensors or actuators, or they may generate data in conjunction with intelligent components. This topic is particularly relevant and interesting for intelligent systems, as edge AI makes intelligent systems even smarter."
For whom and why is Edge AI relevant?
„Edge AI is relevant for many areas of application and stakeholders, not only in industrial context, but also for day-by-day consumer needs. In particular, the automotive, mechanical engineering, health and energy sectors should be mentioned here. Currently, Edge AI is most prevalent in the mobility sector if you think of intelligent lane changing, parking or autonomous driving. Autonomous agricultural machinery often runs at edge of a Cloud-Edge-IoT infrastructure. Computer vision is used for recognition of weeds or plant diseases using AI and machine learning.
Another application is predictive maintenance and quality assurance in production. For example, machine wear can be detected automatically. There is also great potential for using Edge AI in the renewable energy sector. Here, extremely flexible energy supply/dispatch methods are required. Adjusting the amount of electricity fed into the grid is highly complex and requires a real-time AI enabled response. A human is not capable of such a fast reaction. Another area where new methods are being explored is healthcare. For example, Edge AI is applied to wearable devices and sensors, such as those used in diabetes. In the future, even pacemakers could be controlled by AI.
The first important benefit of Edge AI is latency. This can be illustrated using the example of computer vision for autonomous driving. This requires real-time analysis of which objects, people, cyclists and cars are in the area. It would take too long to make a request to a remote infrastructure and wait for a response from a cloud in a remote data centre.
The second benefit is even more important in terms of the volume of data. There are applications where you have enough time to send the data to the cloud, but the problem is that the networks require a lot of energy to transmit the data. So research and development is moving towards pre-processing data at the edge, where the data is actually collected. This way, only a fraction of the data needs to be sent to the cloud, making it possible to react quickly to different situations in real time and saving energy. Europe needs energy-efficient approaches to data processing, but also for data transmission and storage.
There are also privacy benefits. For example, if I use a device to monitor my health, the data is stored locally and therefore protected. But data security is a challenge. The distributed systems also mean that there are more points of attack.“
What is the contribution of EPoSS to Edge AI?
"With EPoSS, we participated in a CSA called 'Unlock Cloud-Edge-IoT (CEI)'. We identified areas of innovation in which Cloud-Edge-IoT should be further developed, including, of course, Hashtag#EdgeAI. We analysed which players are leading in this area and where European companies can find business opportunities. To validate and discuss the findings, we worked closely with the Edge AI working group at EPoSS. In Europe, the distinction between software and hardware is still very strong. The transition is already underway, but the main competitors are in the US.
Edge AI is very complex and requires specific know-how and engineering tools. At the moment, we are looking at where the European research landscape is, in particular the research landscape supported by the Chips JU. From a competitive perspective, Europe should also seize the opportunity and position itself well in low-power and real-time edge AI. We are trying to help identify and exploit this potential."
Share on